In today’s dynamic financial landscape, the fusion of Machine Learning (ML) and stock prediction unveils a realm of unprecedented possibilities. Let’s embark on an exploration of the transformative applications and key insights that define this captivating domain.
🎯 Use Cases Explored:
Algorithmic Trading:
- Application: Develop automated trading strategies leveraging ML algorithms to analyze historical market data and real-time signals.
- Benefits: Enable adaptive trading decisions, optimize portfolio management, and capitalize on fleeting market opportunities.
- Hashtags: #AlgorithmicTrading #AutomatedInvesting #TradingStrategies
Risk Management:
- Application: Employ ML models for risk assessment, portfolio optimization, and proactive risk mitigation.
- Benefits: Enhance decision-making processes, minimize financial exposure, and safeguard against market downturns.
- Hashtags: #RiskManagement #PortfolioOptimization #FinancialRisk
Sentiment Analysis:
- Application: Utilize ML techniques to analyze market sentiment from social media, news articles, and financial forums.
- Benefits: Gain insights into investor sentiment, anticipate market trends, and make informed investment decisions.
- Hashtags: #SentimentAnalysis #MarketSentiment #InvestorBehavior
Anomaly Detection:
- Application: Deploy ML algorithms to detect irregular patterns and anomalies in financial data, aiding in fraud detection and market surveillance.
- Benefits: Identify fraudulent activities, mitigate operational risks, and maintain market integrity.
- Hashtags: #AnomalyDetection #FraudDetection #MarketSurveillance
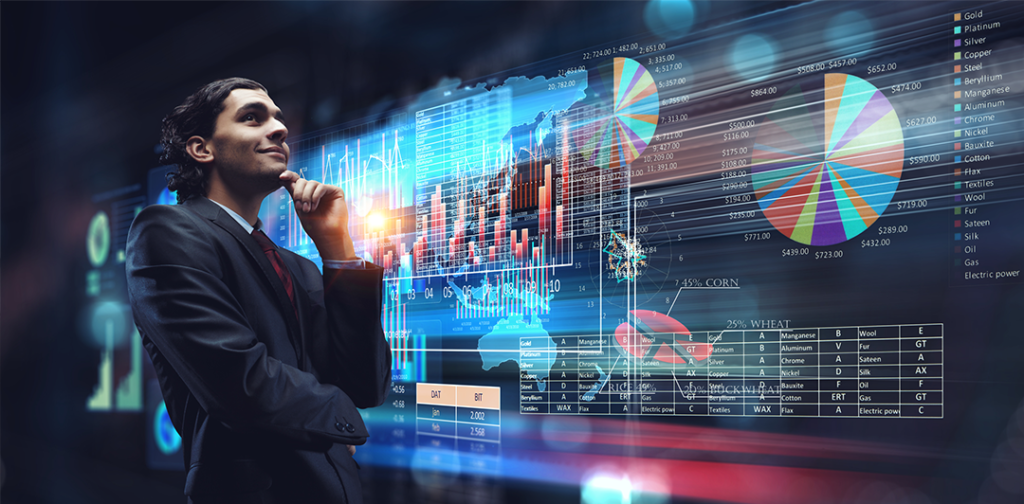
🔑 Key Insights:
Data Quality Matters: Robust ML models hinge on high-quality, comprehensive datasets. Clean, reliable data is indispensable for accurate predictions and actionable insights.
Feature Engineering: Crafting informative features from raw financial data is pivotal for model efficacy. Incorporating technical indicators, economic metrics, and sentiment analysis enriches predictive capabilities.
Model Selection: Experimentation with diverse ML algorithms such as Random Forests, LSTM Networks, and Gradient Boosting Machines aids in identifying the optimal model for specific stock prediction tasks.
Continuous Learning: The dynamic nature of the stock market necessitates regular model retraining with updated data. Continuous learning ensures adaptability to evolving market conditions and sustains predictive accuracy.
Risk Management is Paramount: While ML empowers predictive analytics, prudent risk management strategies remain indispensable. Effective risk mitigation mechanisms are essential for preserving capital and fostering long-term financial resilience.
Join us on this exhilarating journey into the convergence of ML and stock prediction, where innovation meets opportunity and insights pave the path to financial success! #MachineLearning #StockPrediction #FinancialAnalytics #InvestmentStrategies
Related articles

Optimize Staffing and Recruiting with A/B Testing
A/B testing helps recruiters determine which job postings, candidate communications, and recruitment marketing campaigns attract top talent. This data-driven approach helps recruiters allocate resources without wasting time and money on ineffective strategies. A/B testing helps recruiters improve hire quality, reduce time-to-hire, and streamline their processes.
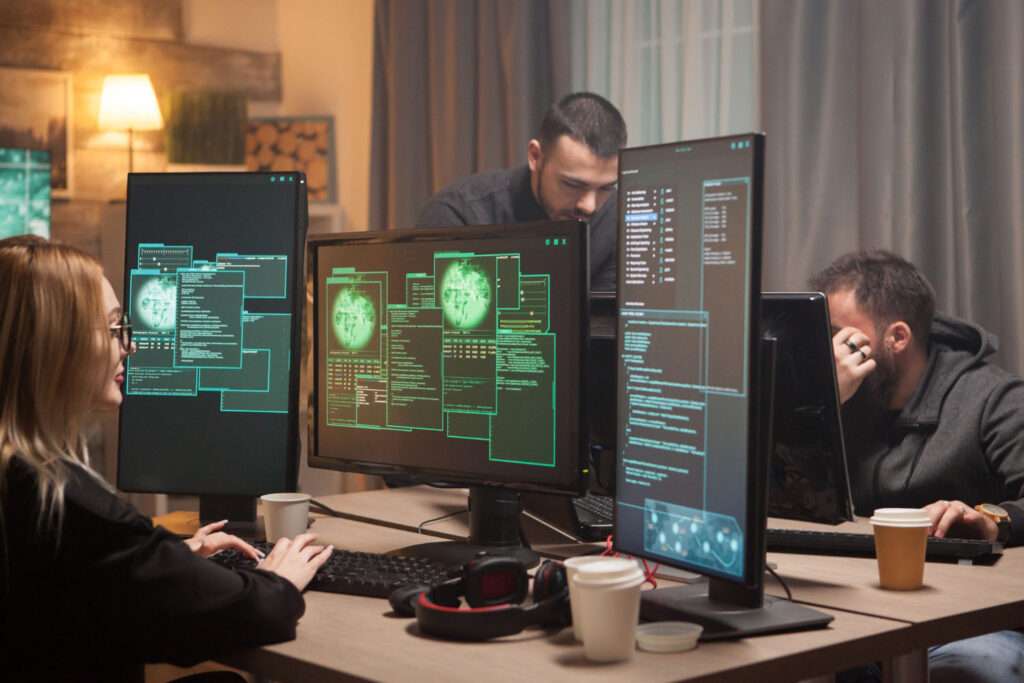
How significant are Linux commands for SOC Analysts?
As a SOC Analyst, having a solid understanding of Linux commands is crucial for several reasons. Linux is a widely used operating system, and many logs are stored in Linux-based systems. Familiarity with Linux commands helps analysts to efficiently navigate and analyze log files, which is essential for incident response and threat hunting. In this article, we’ll explore the essential Linux commands that every SOC Analyst should know, from basic navigation to advanced scripting and automation.

UX & UI Fundamentals
Websites, mobile apps, and software need UX and UI. Look at their basics: User Experience (UX) User Experience (UX) User Experience (UX) Focuses on the